LLMs that “store knowledge” — thoughts from a clinical learning perspective
Some brief, off-the-cuff thoughts around this tweet I came across a few weeks back, specifically regarding of how I utilize knowledge and context together in a clinical setting —

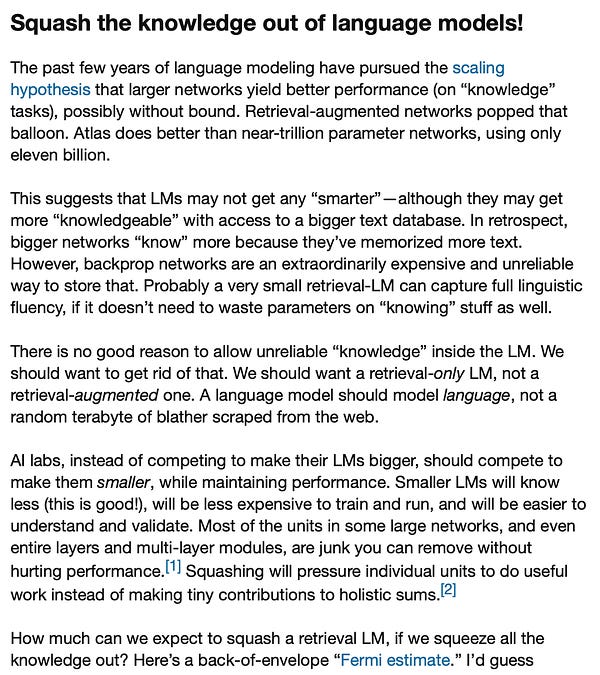
When seeing patients and thinking about treatment plans, a lot of the knowledge I lean on is “database”-type knowledge — or facts that I learned earlier during my first two years of med school.
These include things like the specific pathophysiology of a particular condition, how a medication exerts its effects, clinical indications or contraindications towards a particular treatment
However, other knowledge is purely contextual — for example, components of inpatient medicine like how to transition a patient from an inpatient medication regime to outpatient schedule is dependent on a lot of individual factors. While there are broader rules around this, I feel like I learned a significant portion about this particular topic from seeing it be done many times over.
In some cases, delineating between “database” knowledge and “contextual” knowledge is tricky — I may not explicitly remember (at the time) why a particular treatment option is indicated; however, because I have seen it being used multiple times for similar clinical scenarios, I have an inkling of where to being my thoughts, and follow up with more research on that topic
One example is the use of maintenance fluids, as I was starting my Internal Medicine clerkship — at the beginning of the rotation I didn’t have a clear idea of what type of fluids to use in what scenarios, but seeing many real-world instances repeatedly gave me a good starting point to being understanding the theory
Intuitively, it seems like “database” knowledge is relatively “inexpensive” in the sense that there already exist structured clinical guidelines, algorithms, and tables which can be easily accessed by models. However, patterns learned through seeing examples repeatedly are much more “valuable”. There may be a significant overlap between “database” knowledge and “contextual” knowledge, but working to make models smaller for the sake of efficiency may result in losing some precious contextual knowledge which may not be obtainable elsewhere.